Natively Python
Seamless integration of John Snow Labs' ecosystem with common Python libraries.
Powerful One-Liners
10,000+ models in 250+ languages are at your fingertips with one line of code.
Open Source
Open Source software, widely deployed, back by an active community.
Powered by the most widely used NLP library in the enterprise
Gradient Flow NLP Survey, 2021.
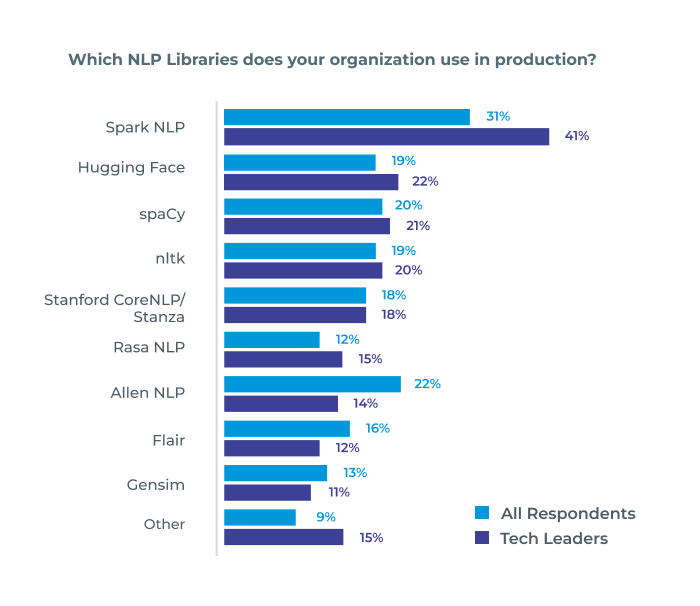
111
!pip install johnsnowlabs
nlp.load('ner').predict("Dr. John Snow is a British physician born in 1813")
entities | entities_class | entities_confidence | |
---|---|---|---|
0 | John Snow | PERSON | 0.9746 |
0 | British | NORP | 0.9928 |
0 | 1813 | DATE | 0.5841 |
nlp.load('sentiment').predict("Well this was easy!")
entities | entities_class | entities_confidence | |
---|---|---|---|
0 | Well this was easy! | pos | 0.999901 |
Complete NLP Ecosystem
Spark NLP
Open-Source text processing library for Python, Java, and Scala. It provides production-grade, scalable, and trainable versions of the latest research in natural language processing.
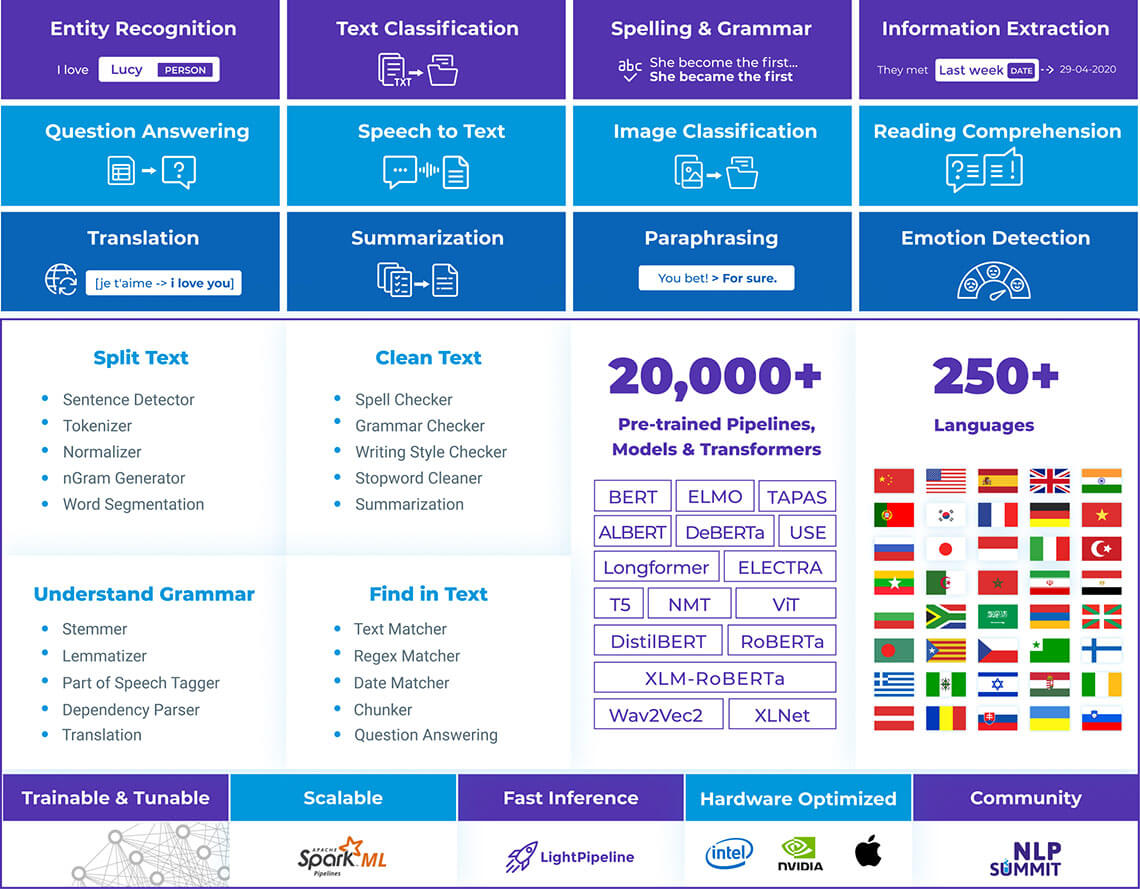
NLP Display
Open-Source Python library for visualizing the annotations generated with Spark NLP.
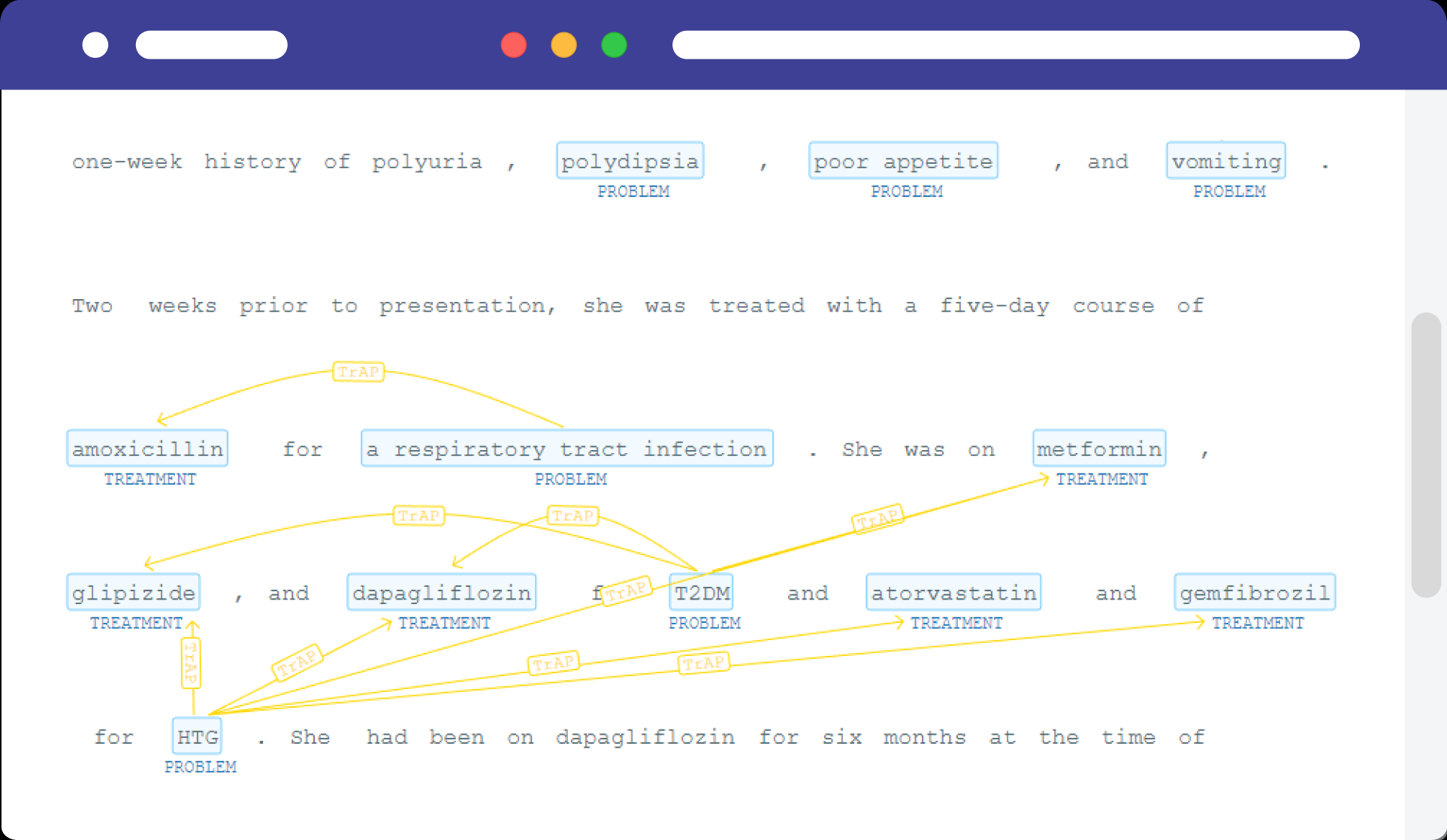
NLP Test
Deliver safe and effective models with an open-source library by generating & running over 50 test types on the most popular NLP libraries & tasks.
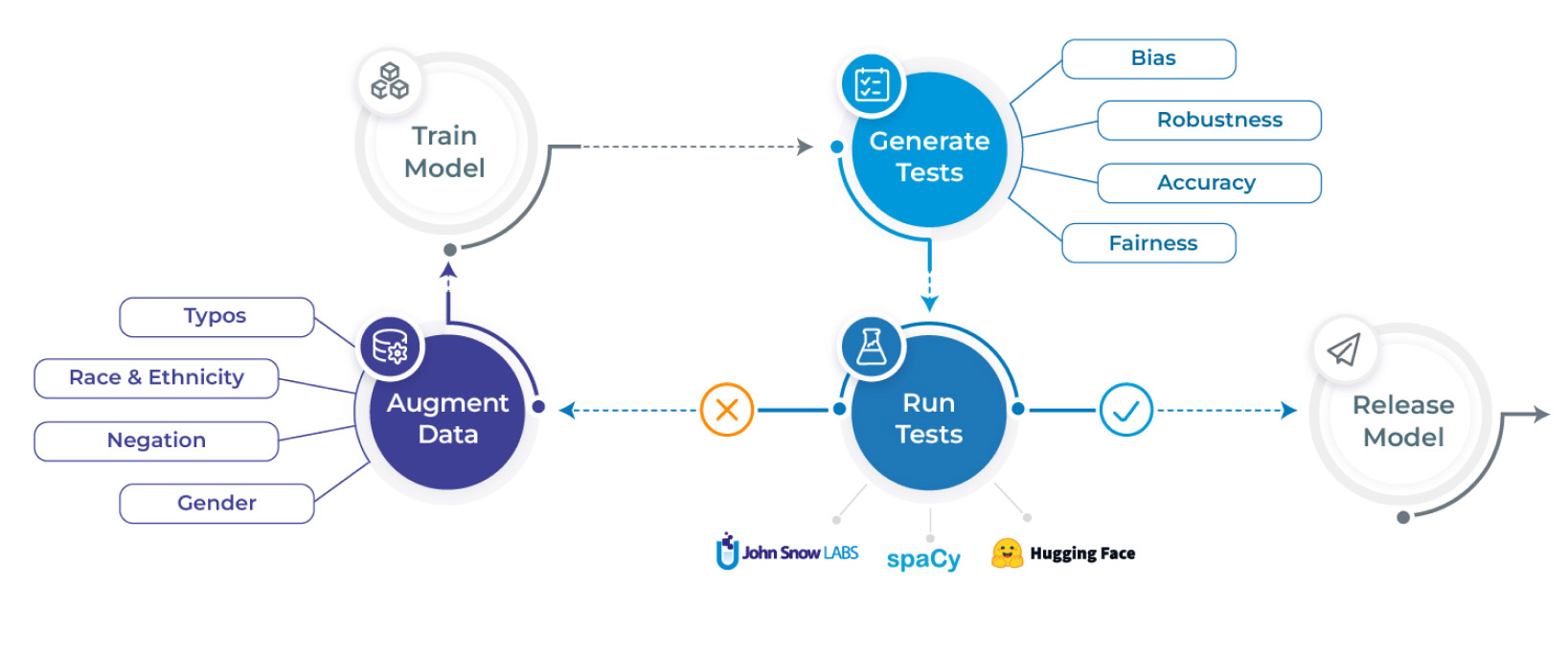
Visual NLP
Visual document understanding library for extracting information from images, scanned documents, PDFs, DOCX, and DICOM files.
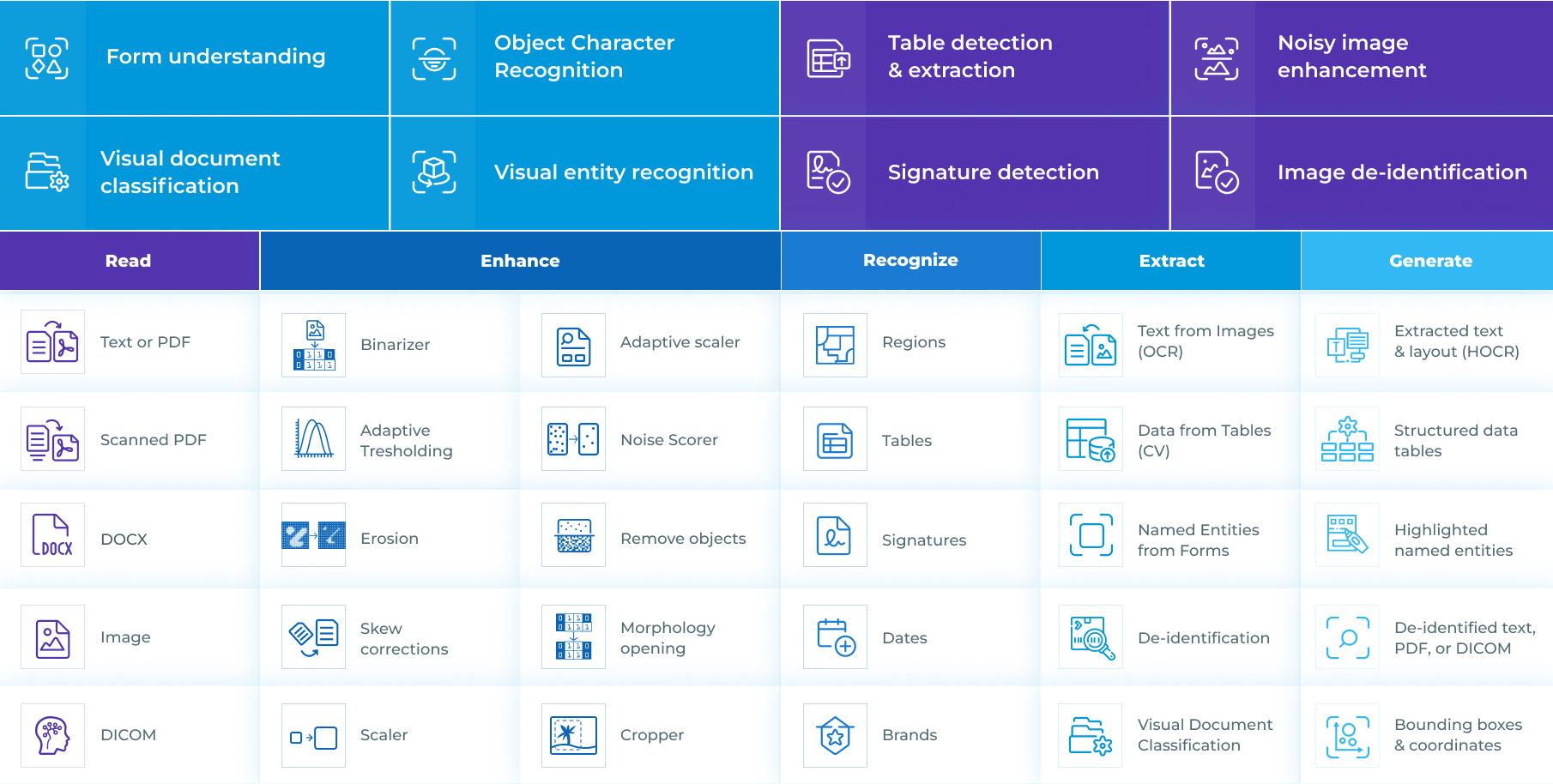
Trusted By














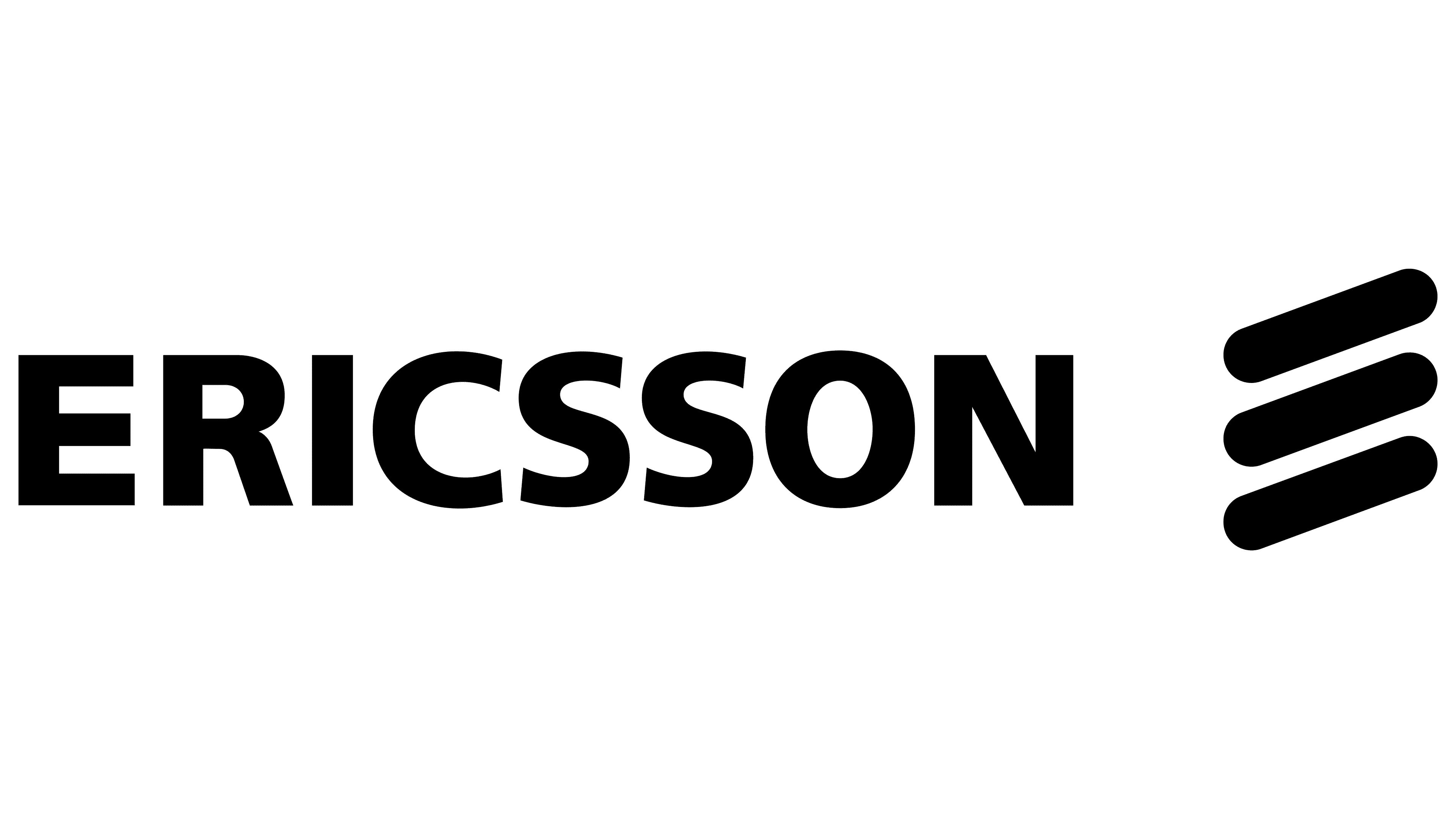


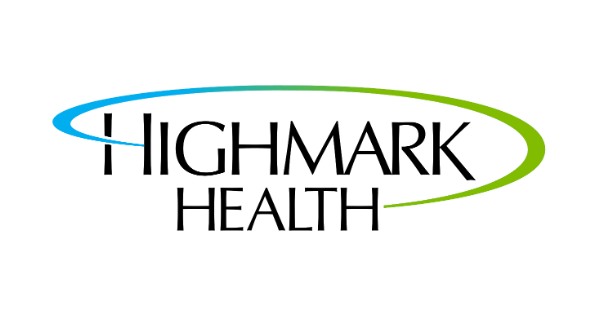









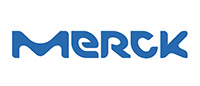


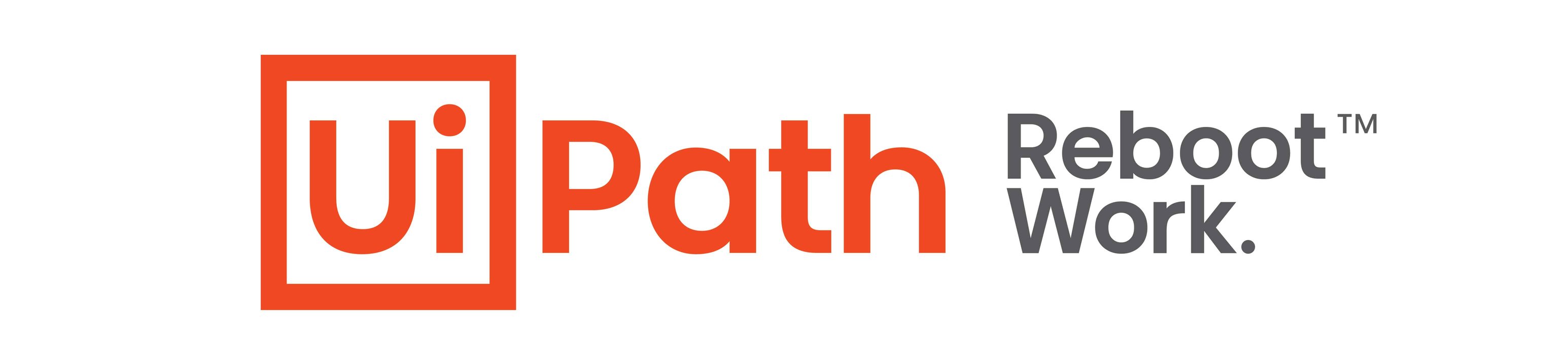
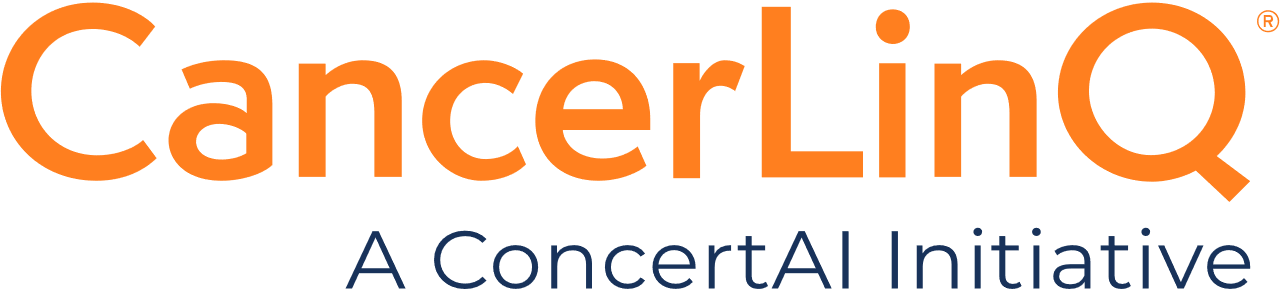




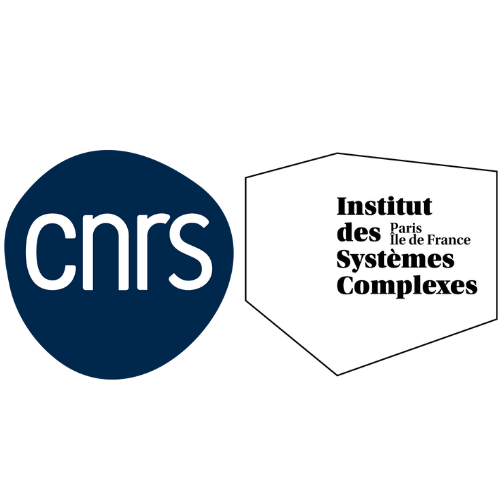



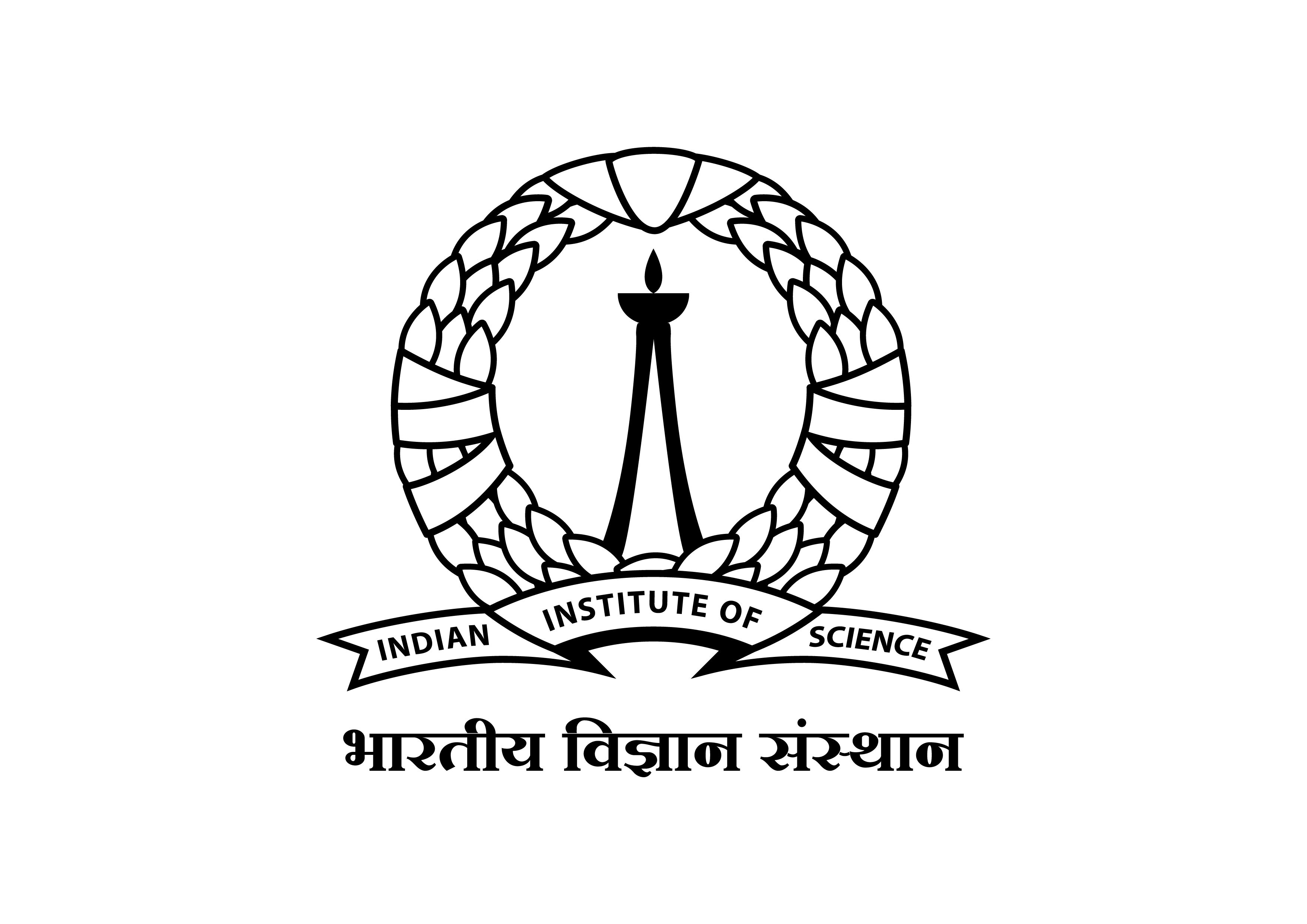
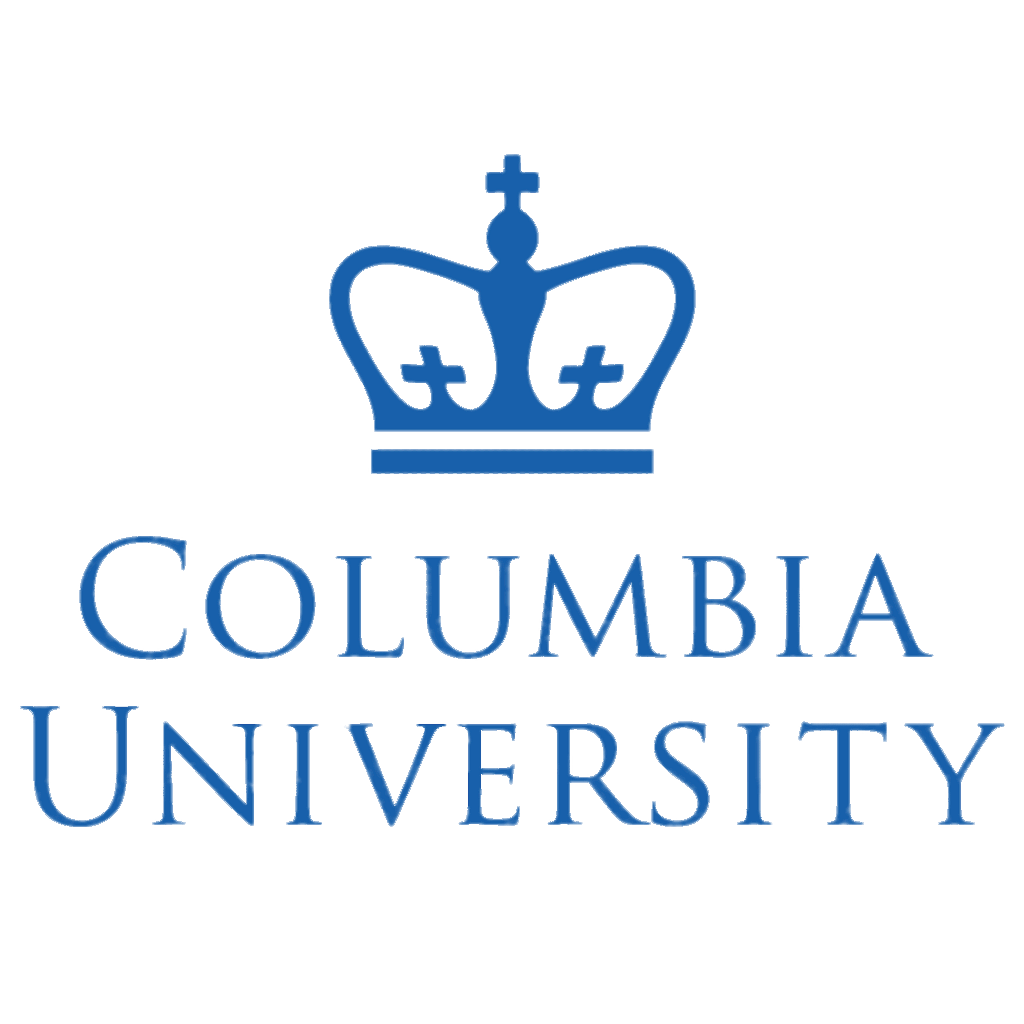
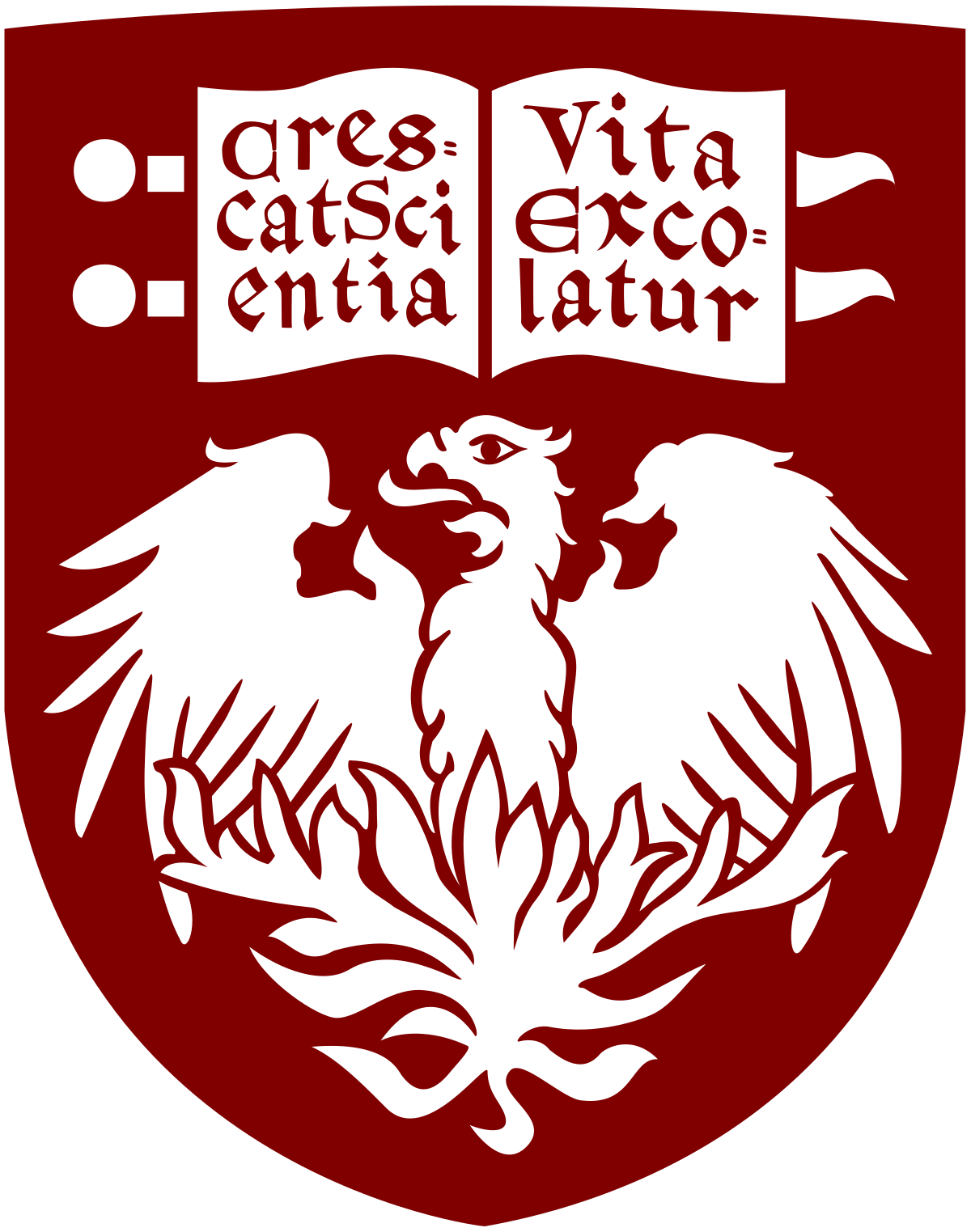

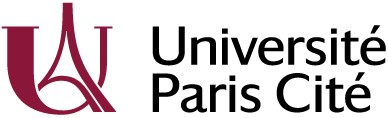
